Quantum machine learning represents a groundbreaking convergence of quantum computing and artificial intelligence, poised to revolutionize data processing and predictive modeling. As quantum computers evolve, their ability to handle complex calculations exponentially faster than classical computers opens new possibilities for machine learning, making it more efficient and powerful. But why is this transformation happening now, and how are industry leaders already harnessing its potential? In this article, we delve into the mechanics of quantum machine learning, explore its practical applications, and highlight the skills needed to thrive in this rapidly advancing field, while also addressing the challenges and future directions of this emerging technology.
gameslino.com invites you to explore this topic thoroughly.
1. Why Quantum Machine Learning?
Quantum machine learning (QML) emerges as a pivotal innovation because it addresses limitations inherent in classical machine learning models. Traditional approaches, while powerful, often struggle with processing vast, complex datasets and optimizing algorithms for tasks like pattern recognition, predictive analytics, and decision-making. As data grows more intricate and voluminous, classical systems can become bottlenecks, limiting their ability to deliver real-time insights.
Quantum computing, with its ability to process multiple possibilities simultaneously through quantum bits (qubits), offers a transformative solution. QML leverages quantum principles such as superposition and entanglement to enhance the speed and accuracy of machine learning tasks. By enabling more efficient handling of large datasets and complex computations, quantum algorithms can dramatically reduce the time required to train models, making it possible to solve problems previously considered intractable.
Moreover, QML opens new frontiers in areas like drug discovery, financial modeling, and artificial intelligence, where traditional methods fall short. The integration of quantum computing into machine learning not only promises to accelerate the development of advanced algorithms but also to unlock new levels of optimization and innovation across industries. This makes QML not just a compelling advancement but a necessary evolution for industries striving to maintain a competitive edge in an increasingly data-driven world.
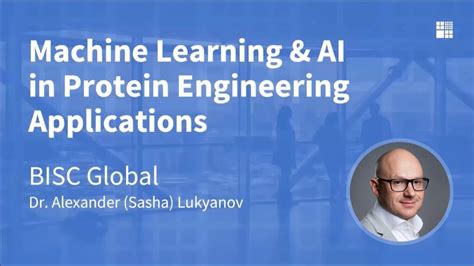
2. Why Now?
Quantum machine learning is gaining momentum now due to a confluence of technological advancements, increased data complexity, and the growing limitations of classical computing. The rapid development of quantum hardware, such as more stable qubits and improved error correction, has brought us closer to practical quantum computers capable of handling real-world problems. Additionally, the exponential growth in data generation has outpaced the capacity of traditional computing systems, creating an urgent need for more powerful processing methods.
Moreover, industries across sectors are under pressure to innovate and optimize their operations, driving demand for faster and more accurate machine learning models. Governments and major tech companies are heavily investing in quantum research, leading to breakthroughs that make quantum machine learning not just a theoretical possibility but an emerging reality. The current intersection of technological readiness and industry demand makes now the opportune moment for quantum machine learning to begin transforming data processing and decision-making on a global scale.
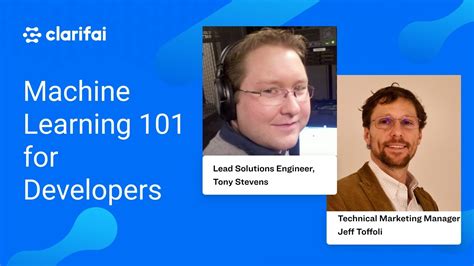
3. How Quantum Machine Learning Works
Quantum machine learning works by combining the principles of quantum computing with traditional machine learning algorithms to create models that can process information more efficiently and accurately. At its core, quantum computing utilizes qubits, which, unlike classical bits that are either 0 or 1, can exist in multiple states simultaneously thanks to superposition. This allows quantum computers to perform many calculations at once, drastically speeding up the processing time for complex tasks.
Entanglement, another quantum property, enables qubits that are entangled to instantaneously affect each other’s state, no matter the distance. This interconnectedness allows quantum algorithms to handle large datasets more effectively, optimizing processes such as pattern recognition, clustering, and classification.
In quantum machine learning, quantum algorithms are used to train models, enhance feature selection, and improve data analysis. These algorithms can exponentially reduce the time required to solve problems that would take classical computers years to compute. By leveraging these quantum properties, quantum machine learning aims to tackle challenges that are currently beyond the reach of classical machine learning techniques.
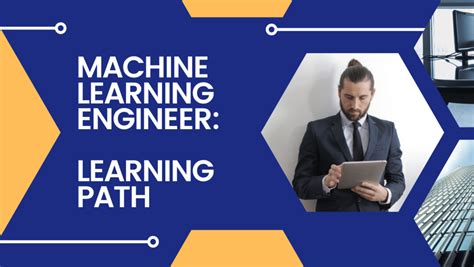
4. How Industry Leaders are Implementing Quantum Machine Learning
Industry leaders are actively implementing quantum machine learning (QML) to gain a competitive edge in solving complex problems and optimizing processes. Tech giants like IBM, Google, and Microsoft are at the forefront, integrating QML into their operations and research. IBM’s Qiskit framework, for example, provides tools for developing quantum algorithms that enhance machine learning models, allowing businesses to experiment with quantum solutions in real-world scenarios.
Google’s quantum computing team has achieved significant milestones, including quantum supremacy, and is now focusing on applying these advancements to machine learning tasks like natural language processing and image recognition. Microsoft’s Azure Quantum offers cloud-based quantum computing services, enabling organizations to develop and test QML models without needing their own quantum hardware.
In the financial sector, companies like Goldman Sachs and JPMorgan Chase are exploring QML for risk analysis, portfolio optimization, and fraud detection. The pharmaceutical industry is also leveraging QML to accelerate drug discovery and molecular modeling, potentially reducing the time and cost involved in bringing new treatments to market. These implementations highlight the growing role of QML in driving innovation and efficiency across various industries.
5. What are the Practical Applications?
Quantum machine learning (QML) offers practical applications across a wide range of industries, providing solutions to challenges that classical computing struggles to address. In finance, QML is used for optimizing trading strategies, improving risk management, and enhancing fraud detection through faster and more accurate data analysis. By processing large volumes of complex financial data, QML enables institutions to make more informed decisions in real time.
In healthcare, QML accelerates drug discovery by simulating molecular structures and interactions at unprecedented speeds, potentially leading to faster development of new treatments. Additionally, it enhances personalized medicine by improving predictive models used in patient diagnosis and treatment planning.
The automotive industry benefits from QML in the development of autonomous vehicles, where it improves sensor data processing and decision-making algorithms, ensuring safer and more reliable self-driving systems.
Moreover, QML’s ability to optimize complex supply chains and logistics makes it valuable for industries like manufacturing and retail, where it enhances efficiency and reduces costs. These practical applications demonstrate the transformative potential of QML in solving real-world problems.
6. What Skills and Knowledge are Needed?
To excel in quantum machine learning (QML), a unique blend of skills and knowledge is required, bridging both quantum computing and machine learning disciplines. A strong foundation in quantum mechanics and quantum computing principles is essential, including an understanding of qubits, superposition, entanglement, and quantum gates. Proficiency in classical machine learning techniques, including algorithm development, data analysis, and model training, is also crucial.
Programming skills are vital, particularly in languages and frameworks used in quantum computing, such as Python, Qiskit, and TensorFlow Quantum. Additionally, a solid background in mathematics, particularly linear algebra, probability, and statistics, is necessary to grasp the complex algorithms and computations involved.
Knowledge of quantum algorithms like Grover’s and Shor’s algorithms, along with experience in developing and testing quantum circuits, will further enhance one’s ability to contribute effectively in this field. As QML is still an emerging area, staying updated on the latest research and developments is key to maintaining a competitive edge in this rapidly evolving domain.
7. What Challenges Remain?
Despite the promising advancements in quantum machine learning (QML), several significant challenges remain. One major hurdle is the current state of quantum hardware. Quantum computers are still in their infancy, with issues such as qubit instability, error rates, and limited qubit connectivity affecting their performance. These technical limitations restrict the practical application of QML and hinder its scalability.
Another challenge is the complexity of developing quantum algorithms that can efficiently leverage quantum computing advantages. Many existing algorithms are still theoretical and require further refinement and validation to be practical for real-world applications.
Integration of QML with existing classical systems poses additional difficulties. Bridging the gap between classical and quantum computing environments requires developing hybrid approaches and tools that can seamlessly integrate with current infrastructure.
Moreover, there is a shortage of skilled professionals with expertise in both quantum computing and machine learning, making it challenging for industries to build and maintain QML capabilities.
Lastly, addressing ethical and security concerns associated with quantum computing, such as potential vulnerabilities in data encryption, is crucial as the technology evolves. These challenges highlight the need for ongoing research and development to fully realize the potential of quantum machine learning.
8. What’s Next for Quantum Machine Learning?
The future of quantum machine learning (QML) is poised for significant advancements as both quantum computing technology and machine learning techniques continue to evolve. Next, we can expect rapid progress in quantum hardware, with improvements in qubit stability, error correction, and scaling, which will enhance the practicality and efficiency of QML applications.
Researchers are focusing on developing more sophisticated quantum algorithms that can address complex real-world problems and integrate seamlessly with classical systems. This includes creating hybrid models that leverage the strengths of both quantum and classical computing.
In addition, the growth of QML will likely drive new use cases across various industries, including pharmaceuticals, finance, and autonomous systems, where it can offer unprecedented insights and optimization capabilities.
Education and training will also advance, producing a new generation of professionals skilled in both quantum computing and machine learning. As these developments unfold, QML is expected to become increasingly integral to solving complex problems and driving innovation in technology and science.
Quantum machine learning is at the forefront of technological innovation, offering transformative potential across industries. As advancements in quantum hardware and algorithm development progress, QML is set to overcome current limitations and unlock new capabilities. By bridging the gap between quantum computing and machine learning, QML promises to revolutionize data processing and decision-making, driving future breakthroughs in technology and science.
gameslino.com